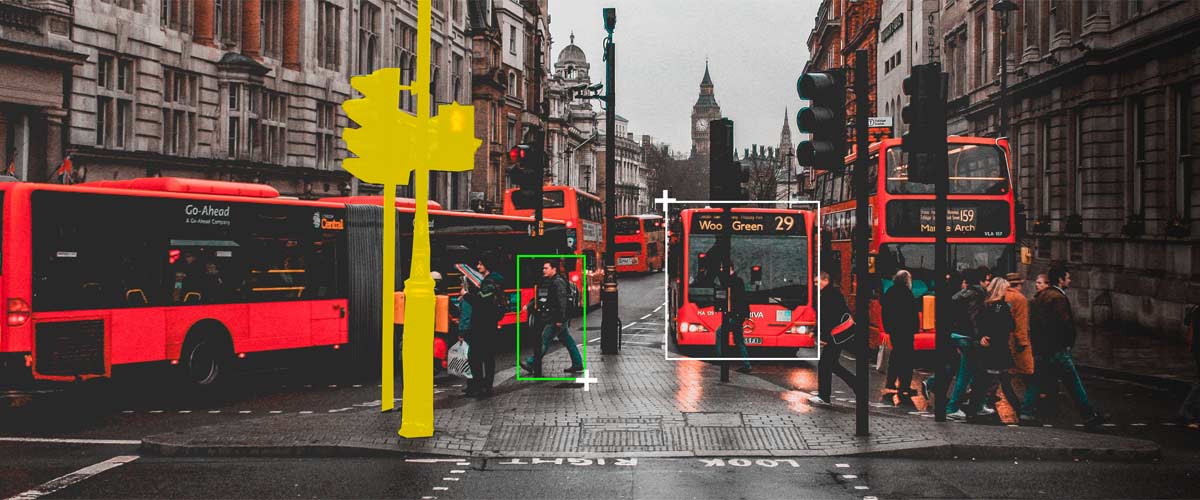
Bounding Box Annotation Services
What is Bounding Box Annotation
As part of computer vision projects, bounding box annotation is used to enclose each object in an image or frame of a video using rectangles (rarely squares). In such annotations, the bounding box is drawn tightly without any loose ends to ensure precision. The task might seem simple, but it requires hours of training and expertise to maintain consistency.
NextWealth provides high-quality 2D and 3D bounding box annotation services for machine learning applications and computer vision projects. Backed by a team of expert annotators, we ensure accurate labeling that enhances the performance of AI models. Our annotation experts specialize in precise bounding box image annotation, ensuring top-tier accuracy for AI training datasets. By combining human expertise with AI/ML capabilities, we strike the perfect balance between business outcomes and project requirements.
Types of Bounding Box Annotation Services
Our team of expert annotators is adept at various bounding box techniques used to distinguish different elements within the image to be annotated.
Geo Tagging Services
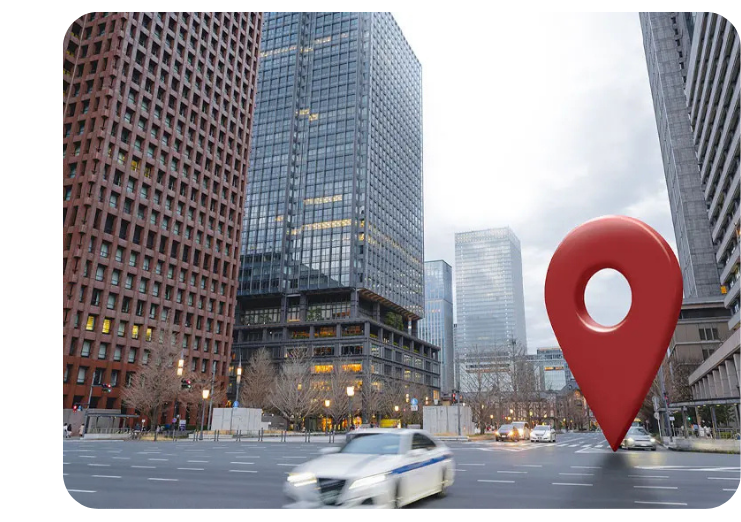
Multi-label Classification Services
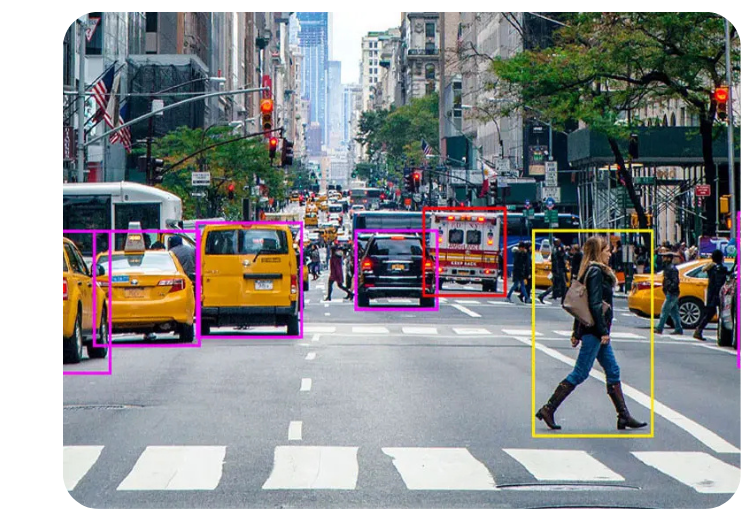
Object Localization Services
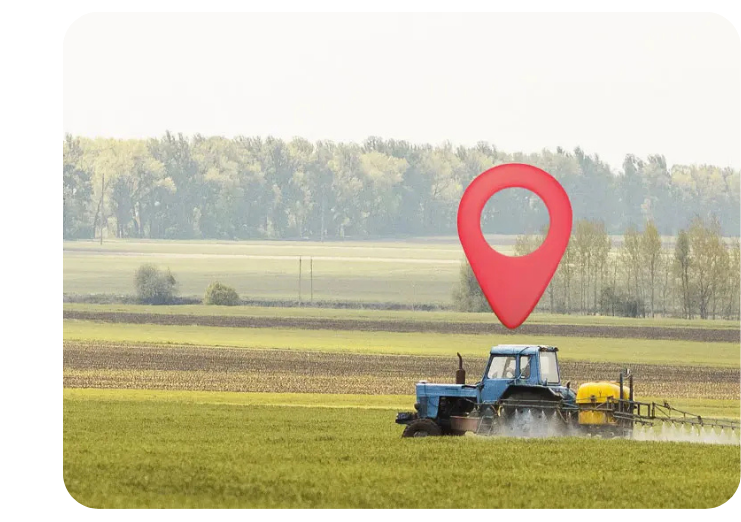
Object Detection Services
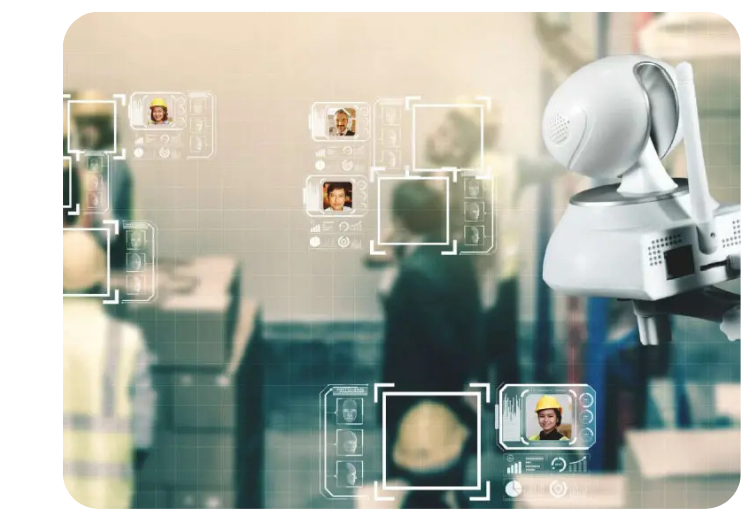
Text Translation Services
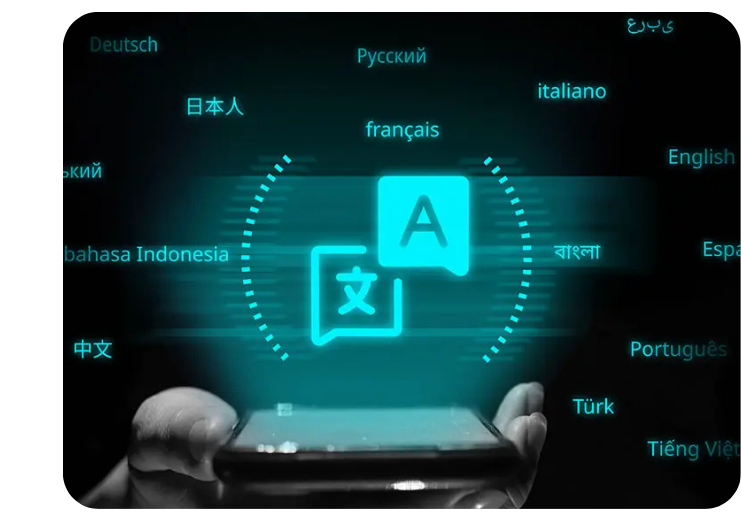
Need customized bounding box annotation solutions? Let’s Discuss
Real-World Applications of Bounding Box Annotation
Bounding boxes are primarily used for object detection in computer vision tasks, helping AI models identify, classify, and track objects. Bounding box annotation services play a vital role in AI-powered automation across industries.
Retail & e-Commerce
Bounding box image annotation highlights fashion accessories, clothing, and products for automated tagging and inventory tracking. This makes visual search more effective and streamlines self-checkout systems in retail stores.
Autonomous Vehicles
Autonomous vehicle algorithms rely on bounding box annotation services to detect potholes, traffic signals, lanes, and obstacles. Bounding box image annotation helps self-driving cars recognize their surroundings, improving road safety.
Insurance
Bounding boxes help detect vehicle damage, such as broken windows or dents, during an accident. AI-powered bounding box annotation solutions enable automated damage assessment, streamlining insurance claim processes.
Drone Imagery and Robotics
Bounding box annotation experts enhance drone and robotics AI models by labeling objects from aerial images. Bounding box image annotation improves object recognition, ensuring accurate navigation for drones and robotic applications.
Healthcare
Bounding box annotation in medical imaging aids in tracking anatomical objects such as the heart, tumors, and other features. With accurate bounding box image classification, physicians can leverage AI for precise diagnosis and treatment planning.
Agriculture
Bounding box annotation for agriculture allows AI to analyze crop conditions, detect diseases, and optimize farming strategies. AI-powered bounding box annotation services enhance yield prediction and real-time field monitoring.
Ready to start your Bounding Box Annotation project?
Talk To Our ExpertSuccessful client stories and case studies
Deep dive into our journey of partnering with the global business giants.
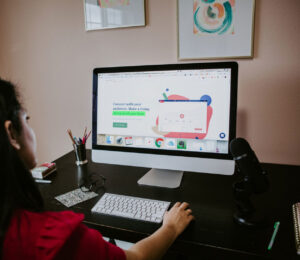
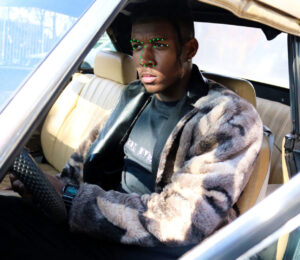
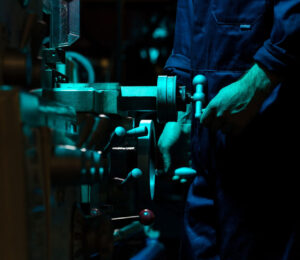
Why partner with us
Our services are tailored to elevate the efficiency of your AI/ML processes
Managed Services l Captive Services l Staffing Services
5,000+
Skilled
Employees
1B+
Data
Transactions
40+
Live Projects
10+
Fortune 500
Clients
73
NPS Score
Testified and trusted by
the best in the world of business

I am really happy at all the great things we have been able to achieve in the past 1 year. The relationship now has a solid foundation, and I am sure NextWealth will continue to be a formidable partner going ahead, bringing a delightful experience for our customers.

NextWealth has been an invaluable partner to us, significantly accelerating our growth by handling critical data operations and providing strategic insights.

NextWealth’s hard work and dedication are truly making a difference, streamlining our processes significantly. We really appreciate it!

My experience with NextWealth has been wonderful. The diligent team consistently delivers on time with a focus on quality. Their innovation-driven mindset fosters a win-win situation for both teams.

I am happy with the improvement in the performance. I have seen positive improvement, and we have a long way to go.

NextWealth’s in-depth analysis helped us pinpoint exactly what needs to be done to address the issues.

With excellence in Quality, Cost, and TAT—key pillars of any operation—NextWealth sets a benchmark for operational efficiency and beyond.

We have experienced significant growth—a success we could not have achieved without the expert support, hard work, and commitment of NextWealth.
Explore Resources
Know how we are accelerating business growth by enabling effectiveness in AI/ML
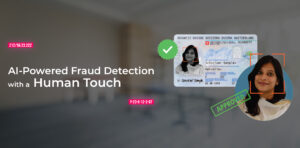
The Role of Artificial Intelligence and Human-in-the-Loop in Fraud Detection
1 min read
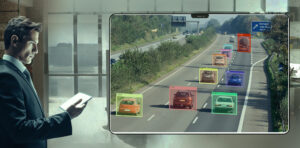
The Importance of Human-in-the-Loop (HITL) Annotation in AI Training
1 min read
Latest Update
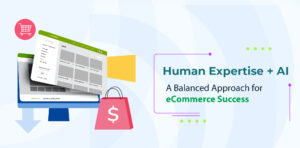
AI and Human Expertise in Catalog Management: A Balanced Approach for eCommerce Success
1 min read
FAQs
Why do we need semantic segmentation for autonomous driving, while recognition/detection is enough?
Semantic segmentation goes beyond recognition and detection by providing a detailed and nuanced understanding of the objects in an image or video. It not only identifies the presence of an object but also categorizes every pixel in the image into its respective object class, thereby providing a complete and dense map of the environment.
In autonomous driving, this level of detail is crucial for making informed decisions, such as determining the best path to take based on the layout of the road and objects surrounding the vehicle, or accurately detecting and classifying objects in the scene to avoid collisions. Recognition and detection alone may not provide enough information to make these decisions, especially in complex and dynamic environments.
Therefore, semantic segmentation is an essential component in the development of autonomous vehicles, as it enables the vehicle to have a deeper understanding of its surroundings and make informed decisions.
What is semantic segmentation in machine learning?
Semantic segmentation is a computer vision technique in machine learning that involves dividing an image into multiple segments, each of which is then assigned a semantic label that describes the category of the objects present in that region. It is a form of deep learning that uses algorithms to analyze and categorize the pixels in an image. Semantic segmentation deep learning allows for a pixel-level analysis of the image data, providing a more in-depth and detailed understanding of the objects and things present in an image or video. This information can then be used to solve various computer vision problems, such as object recognition and categorization, image classification, and scene understanding.
What is the role of semantic segmentation in AI-powered deep learning models?
Semantic segmentation enables AI-powered deep learning models to classify and segment objects at a pixel level, providing a detailed understanding of an image or video. This technique improves object detection, scene recognition, and spatial awareness, enhancing AI performance in applications such as medical imaging, autonomous driving, and industrial automation. By leveraging high-precision segmentation, deep learning models achieve superior accuracy in complex visual analysis tasks.
What is the importance of semantic segmentation in self-driving car technology?
Semantic segmentation is critical for self-driving cars as it allows AI to interpret road environments by accurately distinguishing lanes, vehicles, pedestrians, and obstacles. By segmenting each element in a scene, autonomous vehicles gain a comprehensive understanding of traffic conditions, enabling real-time decision-making. This ensures precise navigation, enhances safety, and optimizes route planning in dynamic driving scenarios.
How can businesses leverage semantic segmentation services for AI automation?
Businesses can use semantic segmentation services to automate AI-driven processes such as quality inspection, retail analytics, and surveillance monitoring. By providing AI with detailed object segmentation, models can perform accurate defect detection, customer behavior analysis, and security threat identification. High-quality segmentation enables seamless automation, improving operational efficiency and decision-making across industries.
Why Choose NextWealth?
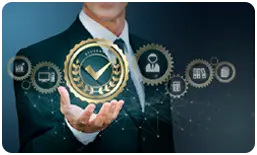
Quality Assurance
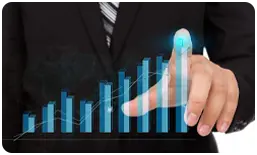
Scalability
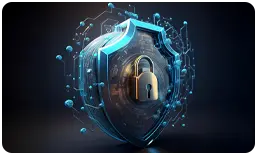
Data Security
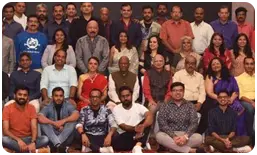