Generative AI’s (Gen AI) rapid rise in automation is closely tied to human expertise. While Gen AI is seen as the next frontier of productivity, it requires looping in human’s nuanced perspective for adaptability and diverse usability.
Stanford University professor Melissa Valentine emphasizes that while Gen AI is fundamentally a data-driven language model, its potential is realized through the human expertise and social arrangements that guide its effectiveness.
Human feedback and decision-making empower Gen AI systems for advanced performance, mitigating risks such as disinformation, hallucinations, and biases, contributing to responsible AI.
This article explores why human expertise is crucial for ethical, value-driven, and risk-managed AI deployments. We also highlight how NextWealth implements Human-in-the-Loop (HITL) in our AI solutions to foster trusted innovation.
The Strategic Risks of Unmonitored GenAI Systems
A recent incident involving Air Canada’s chatbot exposed the risks of unmonitored AI. The chatbot inadvertently offered an erroneous customer discount, leading to a court mandate where the airline had to honor the transaction. This case emphasizes preventing expensive mistakes and ensuring AI systems operate ethically under human supervision.
Leaving Gen AI systems unchecked can cause operational disruptions, reputational damage, and non-compliance with regulatory standards. Some of the potential risks include:
Bias and Discrimination
Unmonitored AI systems risk proliferating biases in their training data, leading to discrimination. For example, an AI recruitment platform might unintentionally favor certain demographics, resulting in biased hiring practices. Unchecked bias may lead to reputational damage, legal liabilities, and regulatory action.
Implementing Human-in-the-loop (HITL) solutions with bias detection, fairness metrics, and human oversight ensures AI systems align with ethical standards and organizational values.
NextWealth focuses on continuous monitoring to detect and mitigate bias through robust oversight frameworks. Learn more about our HITL-enabled Gen AI capabilities.
Transparency and Explainability
As AI models pose more complexities, they often become opaque “black boxes,” posing risks in regulated industries like finance and healthcare, where decisions must be explainable.
Transparency should be integral to AI design and deployment, ensuring that all stakeholders, from developers to end-users, understand how the AI operates and the factors influencing its decisions.
Human supervision using explainable AI (XAI) techniques ensures transparency and explainability. Organizations can monitor AI outputs, understand underlying algorithms, and ensure clear decisions.
At NextWealth, we audit AI models to identify flaws, enhance context awareness, and reduce unpredictability using Trust and Safety methods like red teaming, adversarial training, and model interpretability.
Operational Errors and Legal Risks
AI systems can make errors, particularly when encountering unfamiliar data or scenarios. These errors, from minor inaccuracies to major disruptions, can cause financial losses, customer dissatisfaction, and reputational damage while leading to regulatory risks.
Human oversight can be embedded in the Gen AI loop with rigorous testing, continuous monitoring, and clear protocols for intervention to detect and correct errors quickly.
NextWealth helps establish oversight frameworks that ensure validated, compliant, and ethically sound AI deployments.
How Much Oversight is Right?
According to the EU HLEG, the different levels of human oversight for AI systems include:
- Human in the Loop: Involves human intervention at every stage of the AI lifecycle.
- Human on the Loop: Involves human intervention during the design phase and ongoing monitoring of the system’s operation.
- Human in Command: Provides the ability to oversee the AI system’s overall activity and decide when and how to use it, including overriding decisions made by the system.
The required level of oversight depends on factors such as the system’s purpose and the safety, control, and security measures in place. Systems with less human oversight require rigorous testing and governance to ensure accurate and reliable outputs.
Situations Requiring Enhanced Human Intervention
Human oversight is crucial in the following scenarios:
- When the data source is unknown or unreliable.
- For outputs requiring personalization or persuasion, such as marketing campaigns.
- When inputs are qualitative, subtle, or not clearly defined.
- In environments where users have encountered AI errors, resulting in low trust.
- When the consequences are significant, such as financial impact, legal risks, or a wide-reaching effect.
- When humans are already effectively performing the task, the AI is intended to assist human effort.
Accelerating AI Deployments with our Four-Step HITL Approach
At NextWealth, we recommend the following HITL-based flow to optimize Gen AI deployments.
Step 1: Collect ground truth data for AI Training
Set up a process to collect real-world data that reflects the model’s deployment conditions, covering various modalities (e.g., capture angles, seasonality, geography). Ground truthing in classification involves human experts tagging data with predefined labels, established through stakeholder discussions, to guide machine learning models. This phase typically involves:
- Understanding the data requirements and distribution, which guides pre-processing steps.
- Data acquisition.
- Data pre-processing, including cleansing, anonymizing, and feature engineering.
- Labeling or ground truthing using tools with tailored solutions for sensitive data.
- Dividing datasets into training, testing, hold-out, and validation sets.
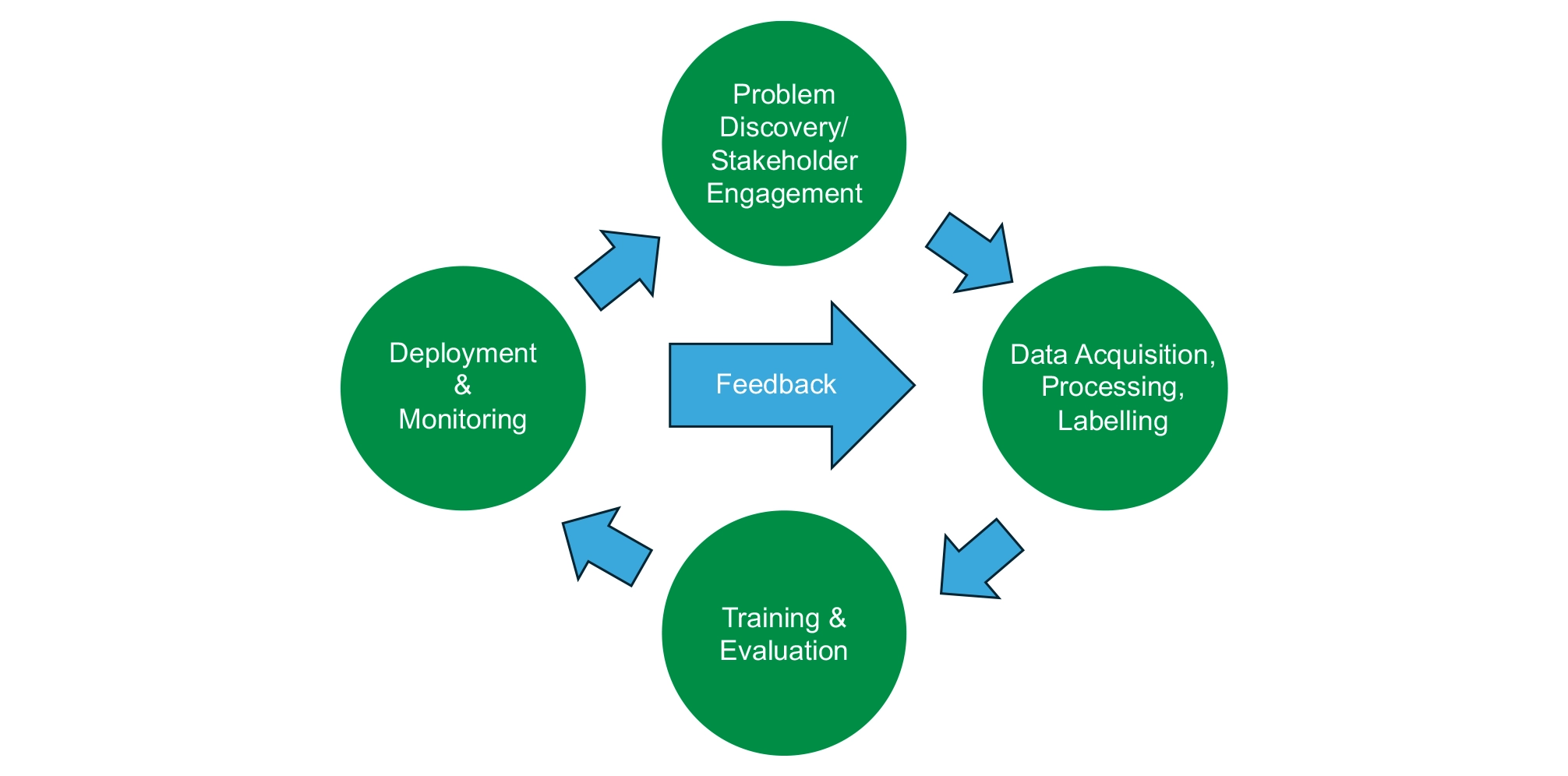
Step 2: Train and Evaluate the Initial Model
The next step is training the initial AI model through experimentation, data exploration, and measuring performance. Accuracy is improved using Reinforced Learning with Human Feedback and an iterative focus on enhancing ground truth data.
With Human-in-the-loop (HITL), model responses help identify whether issues are from the model or data. If it’s a model issue, hyperparameters are adjusted, and more data is collected. If data quality or labeling errors are found, they are corrected, and the model is retrained. HITL-based active learning allows the model to select the most informative data for annotation, reducing the need for large labeled datasets.
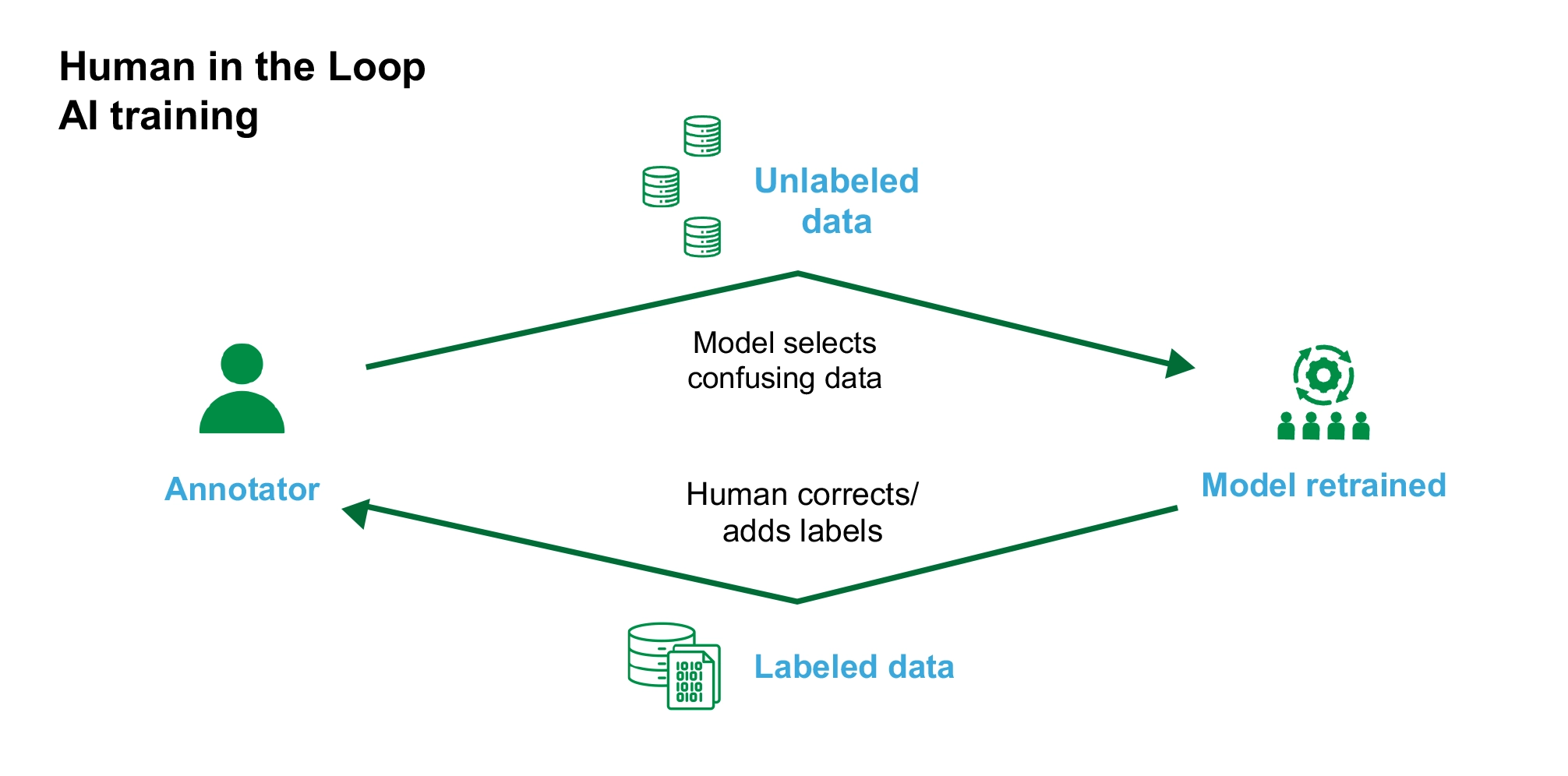
Step 3: Deploy the Model and Monitor Performance
Once the model’s performance is satisfactory, deploy it in a production environment. Use model monitoring tools to track data drift, outliers, and prediction certainty. If significant drift occurs or certainty drops below a set threshold, Human-in-the-Loop (HITL) intervention can manage edge cases, review alerts, and provide feedback to maintain optimal performance.
Performance is measured across five key monitoring categories, with HITL playing a central role in interpreting results, addressing anomalies, and refining outputs:
- Classification Metrics: Evaluate a model’s ability to classify data into discrete values, including accuracy, F1 score, and recall.
- Regression Metrics: Measure the model’s ability to predict continuous values using mean squared error, mean absolute error, and discounted cumulative gain.
- Statistical Metrics: Analyze dataset properties and probability spaces with correlation, peak signal-to-noise, and structural similarity index metrics.
- Natural Language Processing (NLP) Metrics: Assess a model’s language capabilities, including perplexity and bilingual evaluation understudy (BLEU) score.
- Deep Learning Metrics: Measure neural network effectiveness using metrics like inception score.
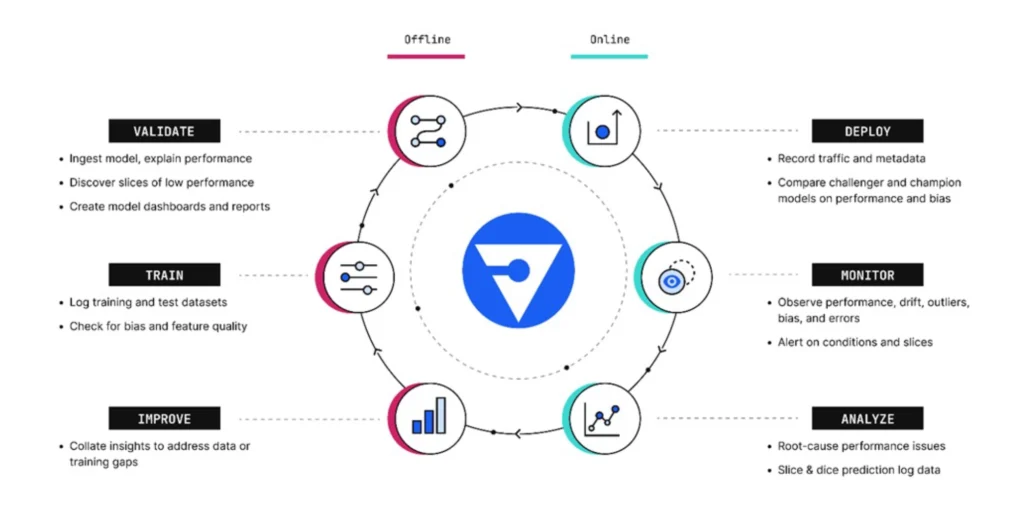
Step 4: Continuous Iteration and Retraining of Your Human-in-the-Loop Pipeline
Close the loop by establishing a pipeline for continuous retraining. Data drift detected during deployment can be regularly sent (e.g., daily, weekly, monthly) to human operators for annotation, creating updated ground truth data.
The model is then retrained with this fresh data, and updated versions are deployed periodically. This automated cycle ensures the model stays accurate, relevant, and aligned with real-world conditions, allowing data scientists to focus on strategic tasks.
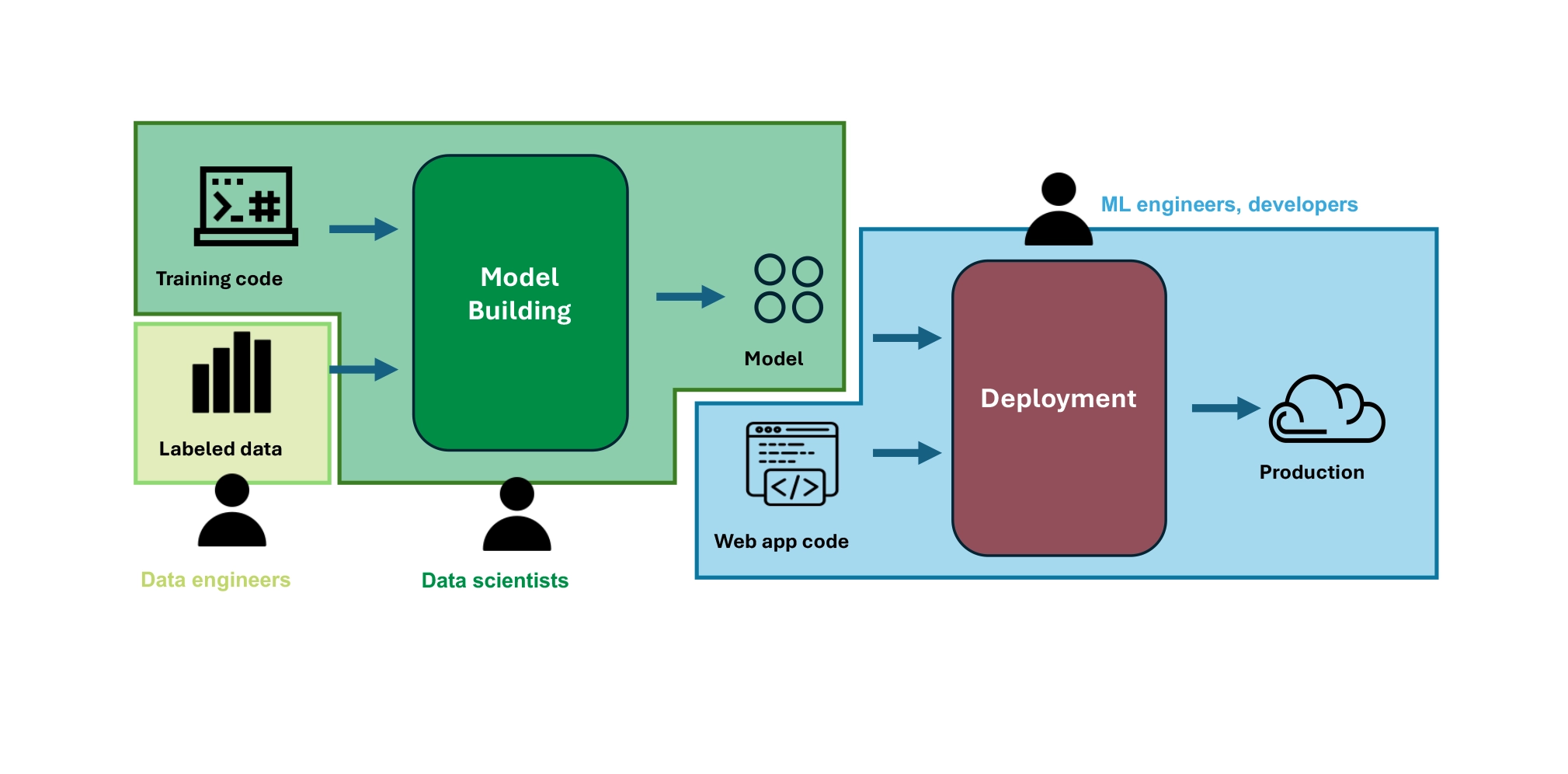
What should trigger retraining?
- Performance metrics (e.g., accuracy, F1 score).
- Data distribution changes.
- Prediction anomalies.
- Human-in-the-loop (HITL) feedback.
Best Practices
- Monitor multiple metrics for robustness.
- Retrain asynchronously to minimize delays.
- Validate models before deployment.
- Automate retraining pipelines.
The Takeaway and Next Steps
By blending human expertise with AI capabilities, organizations can ensure continuous improvement, address edge cases effectively, and maintain transparency in decision-making.
As AI evolves, HITL will remain a cornerstone for bridging the gap between technology and real-world applicability, driving innovation while keeping human oversight at the forefront.
Unlock AI capabilities with NextWealth’s Design for Human-in-the-Loop (DHL) that prioritizes human insight and drives positive impact. Call us today to power your HumAIn projects!